Md Abu Sufian
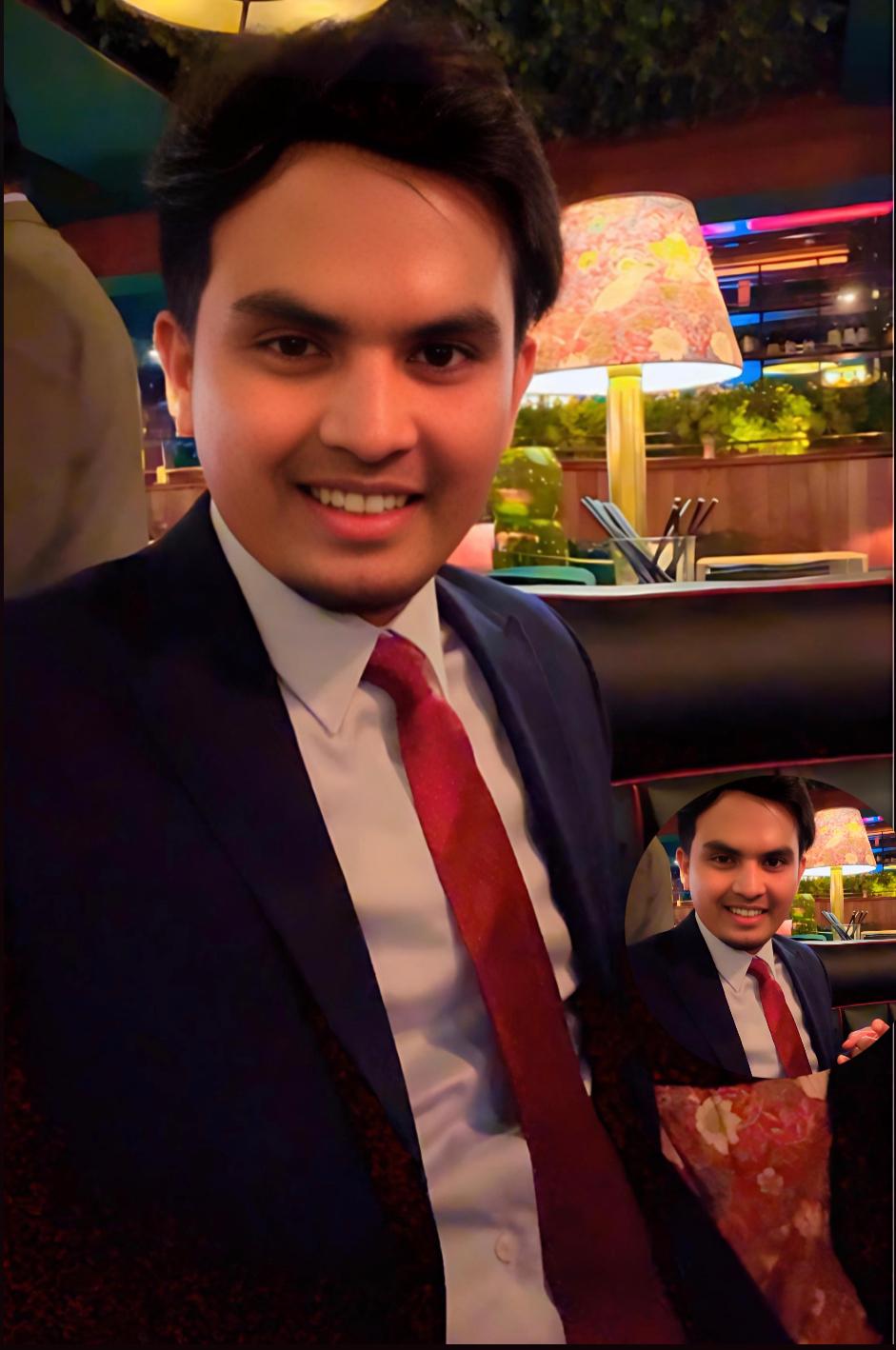
Contact
Email:asufian@kent.edu
Location:London,United Kingdom
GitHub: asufian-github
Linkedin Profile:LinkedIn
ResearchGate:ResearchGate
Orcid ID:Orcid ID
GoogleScholar: GoogleScholar
Sci Profile: Sci Profile
PubMed Profile: PubMed Profile
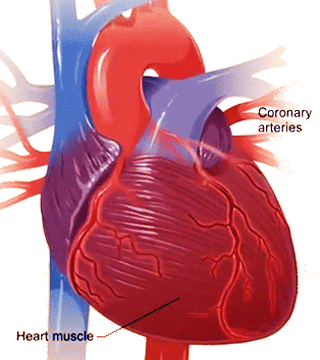
I bring expertise in Bayesian frameworks, statistical analysis, and machine learning, with hands-on experience using TensorFlow, PyTorch, and Scikit-learn. The primary challenge in medical data lies in its high dimensionality, variability, and complexity, which can hinder accurate predictions and insights. To address these issues, I develop advanced AI models integrating multi-modal data sources, including EHRs, genomic information, and medical imaging. I apply deep learning techniques like CNNs and RNNs for robust feature extraction and pattern recognition. By leveraging Bayesian optimisation and advanced statistical methods, I aim to enhance model interpretability and reliability, ultimately developing AI-driven tools for personalised risk assessments and dynamic early warning systems in healthcare. Pursuing a PhD will enable me to refine these approaches further and contribute to transformative advancements in AI-driven healthcare solutions.
In oncology, the primary challenge lies in integrating and analysing heterogeneous data types, including genomic sequences, histopathological images, and clinical records. These datasets' complexity and high dimensionality hinder the accurate prediction of treatment outcomes and patient survival rates. My PhD research will focus on developing advanced machine learning frameworks that effectively handle these data challenges. By leveraging techniques such as deep learning and multi-modal data fusion, I will create predictive models that integrate diverse data sources and provide interpretable insights into tumour behaviour and treatment response. The goal is to develop a robust decision-support system that can assist oncologists in personalised treatment planning, ultimately improving patient outcomes.
Epidemiology faces significant challenges in handling large-scale, longitudinal datasets, often needing help with missing data, bias, and complex temporal dependencies. These issues complicate the accurate estimation of disease prevalence and the identification of risk factors. During my PhD, I will address these challenges by developing novel statistical models and machine learning algorithms tailored for epidemiological data. I will focus on creating methods to handle missing data through imputation techniques, reduce bias via causal inference methods, and accurately model temporal trends using time-series analysis and survival models. The outcome of this research will be the development of a comprehensive analytical toolkit that can provide more accurate and actionable insights for public health interventions.
Cardiovascular data, encompassing EHRs, imaging data, and wearable sensor data, presents challenges regarding data integration, real-time processing, and predictive accuracy. Data quality and noise variability further complicate the extraction of meaningful patterns. My PhD research will focus on developing advanced machine learning models that integrate these diverse data streams, enhance real-time predictive capabilities, and provide personalised risk assessments. Using techniques such as convolutional neural networks for imaging data and recurrent neural networks for time-series data from wearables, I aim to create a predictive system capable of early detection and intervention in cardiovascular diseases, ultimately leading to improved patient care and outcomes.
Data Source: CPRD, UK Biobank
Preprint and Submitted Papers
- Azad, M.A.K., Sufian, M.A., Alsadder, L., Zaman, S., Hamzi, W., Ali, A., Hossain, M.Z., Hamzi, B. (2025). "Hypertension Control in Resource-Constrained Settings: Bridging Socioeconomic Gaps with Predictive Insights" (Q1, Under Review at Elsevier Artificial Intelligence in Medicine, Manuscript ID: AIIM-D-25-00457.
- Sufian.M.A., Niu.,M. (2024)."Advanced Cardiac Electrophysiology Mapping: Precision and Efficiency Using User-Friendly Automated Signal Windowing and Parallel Processing Algorithms Under Review, IEEE Journal of Biomedical and Health Informatics Q1 Manuscript ID: JBHI-02972-2024
- Sufian.M.A., ;Agha. S.;Nora A.M.;Zaman S.,Niu.,M. (2024)."Advancing Alzheimer’s Disease Detection in Clinical Settings: Handwriting and MRI Image Data-Driven Analysis with AI and Deep Learning Techniques Under Review,Q1, Submission ID 2dbf84c1-629e-48ba-980a-54695763b696
- Sufian, M.A.et al.; (2024)."Integrating Machine Learning Strategy for Sustainable Data Management in the UK's Energy Sector: An ESG and GIS Approach Under Review,Q1,Journal of Big Data,Springer,manuscript ID:e70365eb-0802-4383-b4db-b48540508896
- Sufian, M.A. et al.;(2024)."AI and Econometric Analysis of Funding Cuts and Regional Mental Health Disparities in the UK: A Causal and Sentiment-Based Approach for Policy Insights" (1st revision,Q1,submitted at IEEE Transactions on Computational Social Systems,paper No. TCSS-2024-11-1395)
- Hossain, M.F., Sufian, M.A., Miah, M.S., Niu, M. (2024). "Integrating Hydrodynamic Modelling and Data-Driven Approaches for Sustainable Sediment and Flood Management in River Basins" (Q1, Under Review at Environmental Modelling and Software, Manuscript ID ENVSOFT-D-25-00120.
- Sufian, M.A. et al.;(2025)."Different Strategies for the Protection and Analysis of Financial Records: Leveraging Blockchain Technology, Optical Character Recognition, and Advanced Language Models" (Q1,Under Review at EEE Transactions on Knowledge and Data Engineering, Manuscript number RE: TKDE-2025-02-0606)
- Sufian, M.A. et al.; (2023)."AI Models for Early Detection and Mortality Prediction in Cardiovascular Diseases.[Preprint] .
- Islam, M.A., Nag, A., Yousuf, S.M., Mishra, B., Sufian, M.A., Mondal, H. (2024). Preprint | "Data-Driven Analysis: A Comprehensive Study of CPS Case Outcomes in 42 English Counties (2014-2018) with R Analytics" (Preprint at ResearchGate & ResearchSquare)
Announcements and Upcoming Talks in AI, ML, Deep Learning, and Blockchain
Research Paper
- 1) Title: "Advancements in Sensing Technologies for Early Detection of Heart Diseases"
Patent
-
Dual Valve Bioprinting Technology(• Concept: A 3D bioprinting system capable of producing customized, patient-specific valve replacements using their cells. AI ensures precision during the printing and implantation process. • Functionality: If both valves are damaged, printed replacements can restore full heart functionality without the risk of rejection. )AI-Driven Cardiovascular Valve Repair Nanobots( •Concept: Nanobots deployed into the bloodstream that use AI to detect and repair microscopic valve defects. These bots could deposit biocompatible materials or stimulate tissue growth to restore valve function. •Functionality: The nanobots could operate non-invasively and repeatedly as needed, preventing damage progression.)