Md Abu Sufian
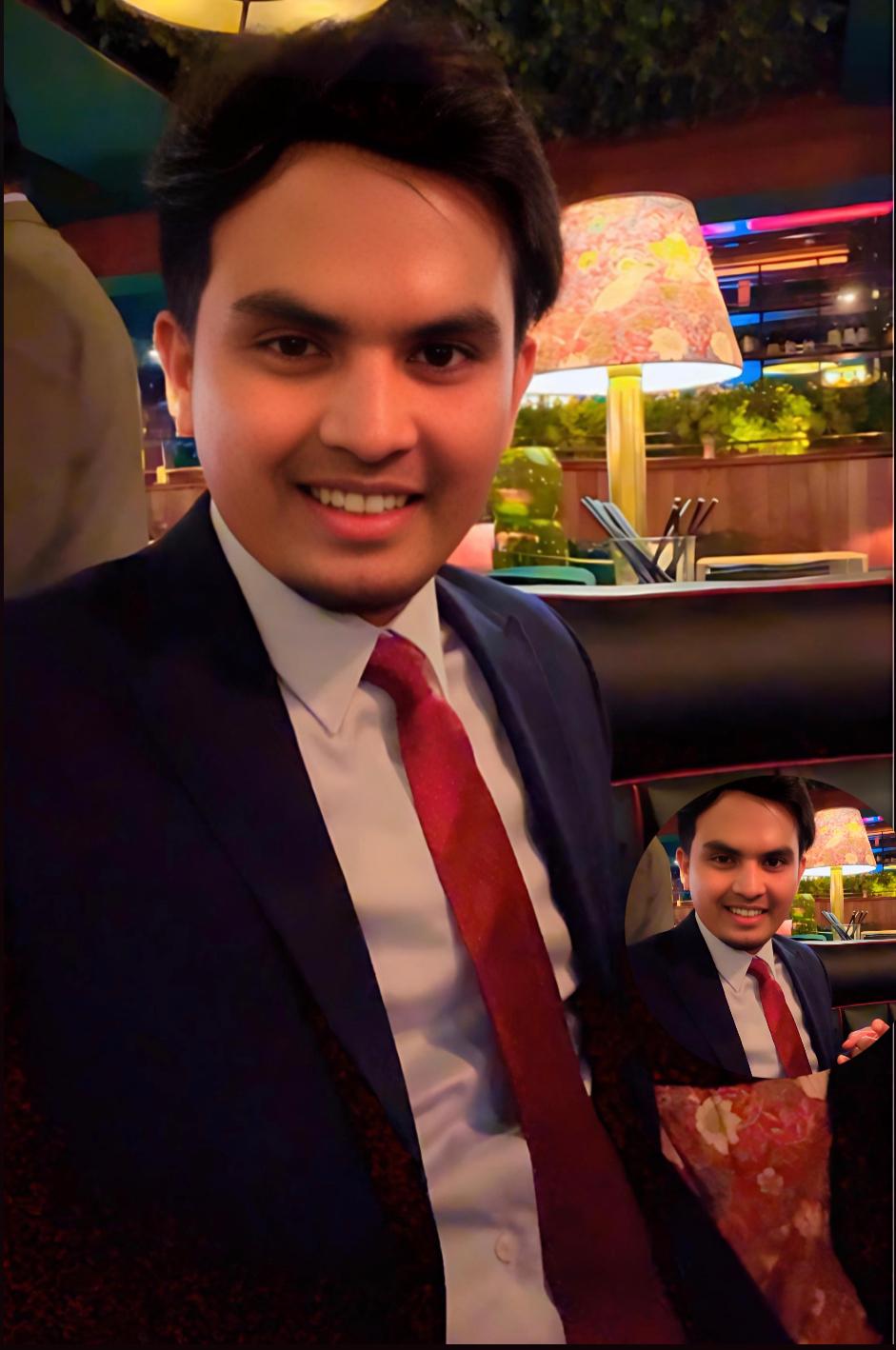
Contact
Email:md.sufian@mail.bcu.ac.uk
Location:London,United Kingdom
GitHub: asufian-github
Linkedin Profile:LinkedIn
ResearchGate:ResearchGate
Orcid ID:Orcid ID
GoogleScholar: GoogleScholar
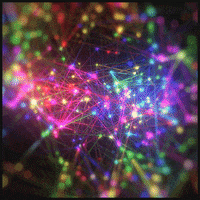
I am seeking a PhD position in advanced machine learning as a skilled data analyst with technical and mathematical expertise. My experience includes diverse skills in Bayesian framework and statistical data analysis, providing high-level insights for decision-making. I have experience using machine learning tools, PowerBI, SQL, and Python as a data analyst in an IT business company and as a graduate research assistant for HSBC Financial Services.
Decisions are based on a combination of factors, such as business knowledge, external factors like market trends and competition, and regulatory requirements, as I have learned from an international MBA program. Machine learning can also aid in quick decision-making processes by identifying complex relationships between sustainable metrics and making predictions based on those relationships, which I learned from the HSBC industry project.
Machine learning includes AI for Health Care: Concepts and Applications, which I learned from Harvard University, fellowship. I gained familiarity with machine learning frameworks and libraries such as TensorFlow, PyTorch, Scikit-learn, and Keras while pursuing my master's in the DABI program. I have a solid grasp of statistical inference, regression analysis, and probability theory and can construct machine-learning models with the aid of data cleaning, feature extraction, and selection. I am familiar with techniques for sentiment analysis, text classification, text generation, and continuous learning methods like neural networks, convolutional neural networks, and recurrent neural networks, as well as optimisation techniques like gradient descent, stochastic gradient descent and especially in Bayesian optimization, where Gaussian processes help in finding the maximum or minimum of a function efficiently.
In my research, I applied machine learning techniques to evaluate the sustainability and risk-weighted assets of companies. This approach not only shed light on their Environmental, Social, and Governance (ESG) performance but also assessed their likelihood of default. My research include data analysis on Electronic Health Records (EHRs), medical imaging, genomic information, laboratory test outcomes, data from wearables and sensors, clinical notes, as well as patient feedback and reviews. I have also developed AI models capable of providing personalized assessments of heart failure risks. These models consider a broad spectrum of factors, including genomic data, lifestyle habits, and existing health conditions, to create highly tailored risk profiles. Additionally, I have worked on creating accessible, AI-powered tools for the general public and developing dynamic monitoring and early warning systems to detect potential health issues promptly.
I am eager to use my expertise in data analysis and machine learning to drive positive change and growth for academia and industry organisations. Pursuing a PhD will allow me to further my understanding of cutting-edge AI,Machine-learning, and Deep Learning techniques in health data science especially in heart related disease as research interest
Preprint and Submitted Papers
- Sufian.M.A., Niu.,M. (2024)."Advanced Cardiac Electrophysiology Mapping: Precision and Efficiency Using User-Friendly Automated Signal Windowing and Parallel Processing Algorithms Under Review, Nature Review Cardiology Q1,Manuscript ID: NRCAR-24-240V1
- Sufian.M.A., ;Agha. S.;Nora A.M.;Zaman S.,Niu.,M. (2024)."Advancing Alzheimer’s Disease Detection in Clinical Settings: Handwriting and MRI Image Data-Driven Analysis with AI and Deep Learning Techniques Under Review,Q1, Manuscript ID: MEDIA-D-24-01115
- Sufian.M.A.; Niu.,M (2024)."Integrating Autoencoders, RNNs, and CNNs for Enhanced Cardiac MRI Analysis via Hybrid Deep Learning: A Multimodal Approach to Precision Diagnostics Under Review, Q1,Computers in Biology and Medicine, CIBM-D-24-067341
- Sufian, M.A.et al.; (2024)."Integrating Machine Learning Strategy for Sustainable Data Management in the UK's Energy Sector: An ESG and GIS Approach Under Review,Q1,Sustainable Management,HELIYON-D-24-32505
- Sufian, M.A. et al.;(2024)."AI-Enabled Study of Funding Cuts in the UK: Exploring Regional Mental Health Disparities through Machine Learning" (1st revision,Q1,Major Revision at Haliyon, Elsevier, Manuscript number HELIYON-D-24-15857R1)
- Sufian, M.A. et al.;(2024)."Different Strategies for the Protection and Analysis of Financial Records: Leveraging Blockchain Technology, Optical Character Recognition, and Advanced Language Models" (Q1,Under Review at Haliyon, Elsevier, Manuscript number HELIYON-D-24-36019)
- Sufian, M.A. et al.; (2023)."AI Models for Early Detection and Mortality Prediction in Cardiovascular Diseases.[Preprint] https://ieeexplore.ieee.org/xpl/RecentIssue.jsp?punumber=6221020.
- Islam, M.A., Nag, A., Yousuf, S.M., Mishra, B., Sufian, M.A., Mondal, H. (2024). Preprint | "Data-Driven Analysis: A Comprehensive Study of CPS Case Outcomes in 42 English Counties (2014-2018) with R Analytics" (Preprint at ResearchGate & ResearchSquare)
Announcements and Upcoming Talks in AI, ML, Deep Learning, and Blockchain
Research Paper
- 1) Title: "An Artificial Intelligence and Machine Learning Model-Based Risk Prediction Calculator of Heart Failure"
- 2) Title: "Enhancing Fairness in Cardiovascular Health: Tackling Algorithmic Bias in Machine Learning Models"
- 3) Title: "Advancements in Sensing Technologies for Early Detection of Heart Diseases"
Patent
-
AI-Enhanced Multimodal Cardiovascular Health Monitoring and Early Detection System(System: This term is broader and can encompass both hardware and software components. A system would integrate devices (hardware) and models (software/algorithms) to provide a comprehensive solution for monitoring and detecting cardiovascular diseases.)